Revolutionizing Robotics: NVIDIA's Groundbreaking AI Tech Unveiled
Revolutionize robotics with NVIDIA's groundbreaking AI tech - open-source model for humanoid robots, leverages simulation, and combines vision-language models for real-time action. A game-changer for helpful robots.
12 tháng 4, 2025
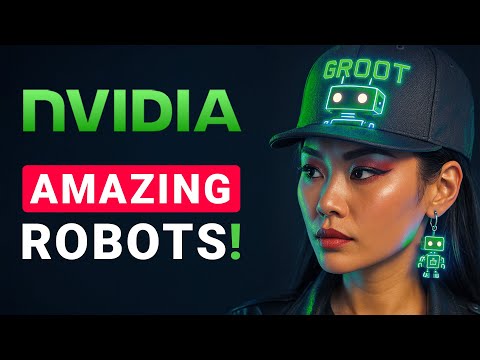
Discover how NVIDIA's groundbreaking AI technology is poised to revolutionize the world of robotics, unlocking new possibilities for helpful and adorable robots that can learn from a wealth of data sources. This cutting-edge research offers a glimpse into the future, where robots can seamlessly integrate into our lives, potentially even saving marriages by taking on household tasks.
The Challenges of Robotics: Data and Labeling Problems
Solving the Data Problem with Simulation and Video Generation
Leveraging Unlabeled Internet Data for Robotics Training
Combining System 1 and System 2 Thinking for Robust Robotics
Diffusion Models and the Robotics Revolution
Limitations and Future Directions
Conclusion
The Challenges of Robotics: Data and Labeling Problems
The Challenges of Robotics: Data and Labeling Problems
Training robots to operate in the real world is an immense challenge due to the lack of readily available and properly labeled data. Unlike language models that can be trained on the vast trove of text data available on the internet, robotics requires data that captures the physical interactions and movements of objects in the real world.
The key problems are two-fold:
-
Data Scarcity: There is a dearth of real-world video data that captures the diverse range of interactions and movements required for robot training. Even with the abundance of videos on platforms like YouTube, this data is largely unlabeled, making it unusable for direct training.
-
Labeling Difficulty: Labeling the data required for robot training is an arduous and time-consuming task. Each video would need to be meticulously annotated with information about the actions, joint movements, and goals of the observed interactions. Scaling this process to the millions of examples needed is simply not feasible.
To overcome these challenges, the researchers have developed a multi-pronged approach:
-
Synthetic Data Generation: By leveraging the Omniverse platform, they can create highly accurate digital simulations of the real world, complete with labeled data on object interactions and movements. This synthetic data can then be further augmented using the Cosmos system to generate realistic video footage.
-
Unsupervised Labeling: The researchers have developed techniques to automatically extract useful information, such as camera movements, joint positions, and action labels, from the vast trove of unlabeled internet videos. This allows them to leverage real-world data as a training resource without the need for manual labeling.
-
Multimodal Integration: By combining the strengths of vision-language models like Eagle-2 with specialized motor control networks, the researchers have created a system that can reason about the world and generate smooth, real-time motor actions. This integration of high-level understanding and low-level control is a key innovation in overcoming the challenges of robotics.
Through these innovative approaches, the researchers have made significant strides in addressing the data and labeling problems that have long plagued the field of robotics. The resulting system, as demonstrated in the paper, shows remarkable improvements in task success rates, paving the way for a new era of capable and helpful robots.
Solving the Data Problem with Simulation and Video Generation
Solving the Data Problem with Simulation and Video Generation
The researchers address the significant data problem that has plagued the field of robotics. Unlike language models that can be trained on the vast amount of text data available on the internet, robots operating in the physical world require labeled data of real-world interactions and movements, which is much more challenging to obtain.
To overcome this, the researchers leverage two key innovations. First, they use a system called Omniverse to create a highly accurate digital simulation of the real world, complete with labeled data on all the movements and interactions. This simulated data can then be used to train the robot models.
However, the researchers found that the simulated data alone was not enough, as it did not always look realistic enough. To address this, they developed a system called Cosmos that can take the Omniverse simulation footage and generate vast amounts of new, realistic videos, while still maintaining the valuable labeled data.
This combination of accurate simulation and realistic video generation allows the researchers to effectively scale up the available training data for the robot models, overcoming the significant data scarcity problem that has plagued the field of robotics. The ability to generate labeled data at scale is a crucial breakthrough that enables the development of more capable and versatile robotic systems.
Leveraging Unlabeled Internet Data for Robotics Training
Leveraging Unlabeled Internet Data for Robotics Training
The researchers have developed a novel approach to address the data scarcity problem in robotics training. By leveraging the vast amount of unlabeled data available on the internet, they have created a system that can automatically extract and annotate relevant information from these videos.
The key components of this approach are:
-
Omniverse and Cosmos: The researchers use Omniverse, a video game-like simulation environment, to generate labeled training data. They then use Cosmos, a system that can take the video game footage as a baseline and create realistic videos, effectively expanding the training data.
-
Self-Supervised Labeling: The researchers have developed a method that allows the AI system to label the unlabeled internet videos by extracting information such as camera movement, joint positions, and actions. This enables the use of real-world data as training material.
-
Vision-Language Model: By building on a previous paper called Eagle-2, the researchers have created a vision-language model that allows the robot to understand the world on two different levels: the slower, reasoning-based System 2 and the faster, real-time System 1.
The combination of these techniques has led to a significant improvement in the performance of the humanoid robotics system, increasing the success rate from 46% to 76% when compared to previous methods. This breakthrough represents a major step towards the development of useful and helpful robots that can assist us in our daily lives.
While the current system is not yet a turnkey solution, the researchers have made the model freely available, allowing the community to fine-tune and improve it for their specific applications. The open and collaborative nature of this work is a testament to the power of scientific progress and the potential for robotics to transform our world.
Combining System 1 and System 2 Thinking for Robust Robotics
Combining System 1 and System 2 Thinking for Robust Robotics
The key to achieving robust and capable robotics lies in the combination of two distinct modes of thinking: System 1 and System 2. System 1 thinking, represented by a diffusion model, enables the robot to generate smooth motor actions in real-time, allowing for immediate and responsive movements. In contrast, System 2 thinking, powered by a vision-language model, provides the robot with the ability to reason about the world, understand its surroundings, and formulate plans.
By integrating these two complementary systems, the researchers have achieved a remarkable improvement in performance, going from a 46% success rate to an impressive 76% when compared to previous methods. This breakthrough represents a significant leap forward, accomplishing in a matter of years what would have previously taken up to a decade to achieve.
The key innovation lies in the use of diffusion models to generate smooth motor actions, akin to how they are used to create images from noise. This novel approach to motion generation, combined with the reasoning capabilities of the vision-language model, enables the robot to understand its environment and execute actions with precision and fluidity.
Moreover, the researchers have made this groundbreaking work fully open and accessible, allowing fellow scholars to build upon it and further refine the technology. This open-source approach paves the way for a robotics revolution, where helpful and capable robots can finally become a reality, potentially even assisting with mundane tasks like laundry folding.
While the current system is not yet a turnkey solution, the researchers have laid the foundation for continued progress. As the technology matures, we can expect to see increasingly sophisticated and versatile robotic systems that can seamlessly integrate with our daily lives, revolutionizing the way we interact with the physical world.
Diffusion Models and the Robotics Revolution
Diffusion Models and the Robotics Revolution
Diffusion models, a technique often used to generate images from noise, have found a surprising application in the field of robotics. Researchers have developed a system that combines a vision-language model, called Eagle-2, with a diffusion-based neural network to create a powerful framework for humanoid robotics.
This approach allows robots to understand the world around them on two levels: a slower, reasoning-based "System 2" and a faster, real-time "System 1" that generates motor actions. The diffusion model, which starts from noise and gradually denoises it, is used to produce smooth, coherent motor actions, rather than just static images.
When compared to previous methods, this new approach has shown a remarkable improvement in success rate, going from 46% to 76%. This breakthrough represents a significant step forward in the field of robotics, bringing us closer to the reality of useful, helpful robots that can perform a variety of tasks.
While this solution is not yet a turnkey solution for home applications, such as folding laundry, the researchers have made the model freely available for others to fine-tune and improve upon. The open and collaborative nature of this work is a testament to the scientific community's commitment to advancing the field of robotics for the benefit of all.
Limitations and Future Directions
Limitations and Future Directions
While the GR00T-N1 paper presents a significant breakthrough in humanoid robotics, the authors acknowledge that it is not a turnkey solution that can be immediately deployed for complex tasks like folding laundry at home. The model is still focused on short tasks involving interactions with objects on a table, and further advancements will be needed to expand its capabilities.
The authors note that the current system is not yet ready for widespread deployment, and it may take an additional one or two papers to reach that level of maturity. However, the open-source nature of the model allows researchers and developers to fine-tune and improve it for their specific applications.
Despite these limitations, the authors are excited about the potential of the GR00T-N1 framework and the opportunities it presents for the future of robotics. The combination of simulation data, unlabeled internet data, and the novel diffusion-based motor control system has already demonstrated significant improvements in task success rates, and the authors believe that this approach will continue to drive rapid advancements in the field.
As the Fellow Scholars have already begun experimenting with the GR00T-N1 model, the authors are eager to see how the community will build upon this work and push the boundaries of what is possible in humanoid robotics. The open and collaborative nature of the research is a testament to the power of scientific progress, and the authors are confident that the robotics revolution they envision is well within reach.
Conclusion
Conclusion
This paper presents a groundbreaking approach to humanoid robotics that has the potential to kickstart a revolution in the field. The key innovations include:
- Leveraging simulation data and a video generation system to create a vast, labeled dataset for training robots, overcoming the data scarcity challenge.
- Developing a novel diffusion-based neural network that can generate smooth motor actions in real-time, complementing the slower but more reasoning-based system.
- Integrating a powerful vision-language model to enable robots to understand the world around them at multiple levels.
The combination of these techniques has led to a significant performance boost, improving success rates from 46% to 76% compared to previous methods. This represents a remarkable advancement that could pave the way for useful, helpful robots to become a reality in the near future.
While the solution is not yet a turnkey deployment, the authors have made the model freely available for the community to fine-tune and build upon. The open-source nature of this work is commendable and will undoubtedly accelerate progress in the field.
As the author notes, the excitement around this paper is palpable, and the community is already exploring various applications and embodiments. The future of humanoid robotics looks brighter than ever, and this paper is a testament to the power of collaborative, open-source research.
Câu hỏi thường gặp
Câu hỏi thường gặp