Unlock the Power of a 32B AI Model: Deepseek R1 Challenger Revealed!
Unlock the Power of a 32B AI Model: Deepseek R1 Challenger Revealed! Alibaba's new 32B reasoning model, qwq 32b, rivals the 671B Deepseek R1. Discover the advancements in reinforcement learning, foundation model pre-training, and agent-like capabilities that make this smaller model a strong contender.
22 марта 2025 г.
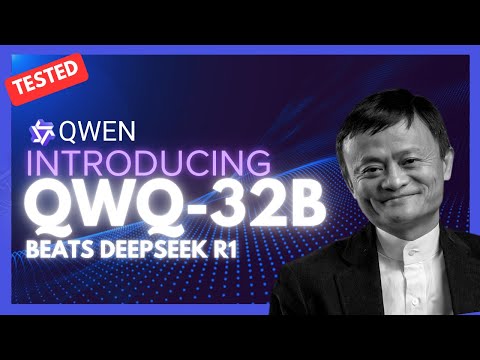
Discover the power of a 32-billion parameter AI model that rivals the performance of much larger models. This blog post explores the impressive capabilities of Alibaba's new QwQ-32B model, which leverages reinforcement learning and advanced techniques to outshine even the 671-billion parameter DeepSeek R1 in various reasoning tasks. Learn how this compact yet mighty model can tackle a wide range of challenges, from web development to logical reasoning, and see why it's a game-changer in the world of AI.
Reinforcement Learning Optimization: How a 32B Model Outperforms a 671B One
Foundation Model Pre-Training: Leveraging World Knowledge for Reasoning
Agent-Like Capabilities: Adaptive Reasoning and Environmental Feedback
Benchmarking the QwQ-32B: Evaluating Reasoning across Tasks
Accessing the QwQ-32B Model: Installation and Try-Out Options
Conclusion
Reinforcement Learning Optimization: How a 32B Model Outperforms a 671B One
Reinforcement Learning Optimization: How a 32B Model Outperforms a 671B One
Alibaba's new 32B parameter model, QWQ 32B, is rivaling top-tier reasoning models like the 671B parameter DeepSEER1 through the power of reinforcement learning optimization. This model achieves high performance by leveraging scaling reinforcement learning, going beyond traditional pre-training and post-training methods.
The key advancements that make this model powerful are:
-
Reinforcement Learning Optimization: RL significantly boosts the reasoning capabilities of the model, making a smaller 32B parameter model more intelligent than the larger 671B DeepSEER1.
-
Foundation Model Pre-training: The model is built on extensive world knowledge, ensuring a strong base for reasoning.
-
Agent-like Capabilities: The model can think critically, use tools, and adapt its reasoning based on environmental feedback.
These three key factors allow the 32B QWQ model to outperform the much larger 671B DeepSEER1 in various reasoning-intensive tasks, as demonstrated by rigorous benchmarking. The model is now accessible through Hugging Face, and you can try it out through Hugging Face Spaces or the Quin chatbot.
Foundation Model Pre-Training: Leveraging World Knowledge for Reasoning
Foundation Model Pre-Training: Leveraging World Knowledge for Reasoning
The qwq 32b model's impressive performance can be largely attributed to its foundation model pre-training, which builds a strong base of world knowledge to enhance its reasoning capabilities. By leveraging extensive pre-training on a vast corpus of data, the model has developed a deep understanding of various domains, from language and common sense to scientific and factual information.
This foundation of world knowledge serves as a powerful starting point for the model's reasoning tasks. When faced with a new problem or query, the model can draw upon its broad knowledge base to make informed inferences, connect relevant concepts, and arrive at more accurate and insightful solutions.
The foundation model pre-training not only provides the model with a solid knowledge foundation but also helps it develop more robust and adaptable reasoning skills. By exposing the model to a diverse range of information and scenarios during pre-training, it learns to think critically, consider multiple perspectives, and adjust its reasoning approach based on the specific context and requirements of the task at hand.
This combination of extensive world knowledge and flexible reasoning capabilities makes the qwq 32b model a formidable tool for tackling a wide range of reasoning-intensive tasks, from mathematical problem-solving to logical deduction and beyond. Its ability to leverage its foundation of knowledge to reason effectively, even with a relatively small parameter count, is a testament to the power of this approach.
Agent-Like Capabilities: Adaptive Reasoning and Environmental Feedback
Agent-Like Capabilities: Adaptive Reasoning and Environmental Feedback
The new reasoning model from Alibaba, the qwq 32b, showcases impressive capabilities that go beyond traditional pre-training and fine-tuning methods. Three key advancements underpin its performance:
-
Reinforcement Learning Optimization: The model leverages reinforcement learning to significantly boost its reasoning capabilities, making a smaller 32 billion parameter model more intelligent than larger models.
-
Foundation Model Pre-training: The model is built on extensive world knowledge, ensuring a strong base for reasoning tasks.
-
Agent-Like Capabilities: The model can think critically, use tools, and adapt its reasoning based on environmental feedback, demonstrating an agent-like approach to problem-solving.
These advancements allow the qwq 32b to compete with and even outperform significantly larger models, such as the 671 billion parameter Deep Seek R1, in various reasoning-intensive benchmarks. The model's ability to excel despite its smaller size is a testament to the power of reinforcement learning and the importance of foundation model pre-training for building capable reasoning systems.
Benchmarking the QwQ-32B: Evaluating Reasoning across Tasks
Benchmarking the QwQ-32B: Evaluating Reasoning across Tasks
The QwQ-32B, Alibaba's new 32-billion parameter reasoning model, has been put through rigorous benchmarking to evaluate its performance across various reasoning-intensive tasks. The tests are designed to measure how well the model performs in areas such as math reasoning, coding, and other capabilities.
When compared to top-tier models like the 671-billion parameter Deep Seek R1, the QwQ-32B holds its own, often matching or even slightly outperforming the larger model. This underscores the effectiveness of the scaling reinforcement learning techniques used in the QwQ-32B, which enhance the model's intelligence and prove that a smaller, 32-billion parameter model can compete with or outperform significantly larger architectures.
The open weights for the QwQ-32B model are now accessible through Hugging Face, allowing users to try it out through Hugging Face Spaces or access it through Model Scope. The model is available under the PyT 2.0 license and can also be accessed through the Quin chatbot.
Overall, the QwQ-32B's performance in the benchmarking tests demonstrates its strong reasoning capabilities, making it a compelling option for a wide range of applications that require intelligent decision-making and problem-solving.
Accessing the QwQ-32B Model: Installation and Try-Out Options
Accessing the QwQ-32B Model: Installation and Try-Out Options
The QwQ-32B model, Alibaba's new 32-billion parameter reasoning model, is now accessible through various channels. Here's how you can get started with it:
-
Open Weights and Hugging Face Spaces: The open weights for the QwQ-32B model are available through Hugging Face. You can try out the model by accessing it through Hugging Face Spaces.
-
Model Scope Access: The QwQ-32B model can also be accessed through Model Scope, as it is released under the Py 2.0 license.
-
Quin Chat Integration: Alibaba has integrated the QwQ-32B model into their Quin chatbot. You can interact with the model directly through the Quin chat interface.
-
Local Installation with LM Studio: You can install the QwQ-32B model locally using LM Studio. This allows you to experiment with different model sizes and configurations.
-
AMA Model Installation: The QwQ-32B model is also available for installation through AMA. You can visit their model site and easily set up the model on your system.
By leveraging these various access points, you can explore the capabilities of the QwQ-32B model and assess its performance across different reasoning-intensive tasks. The model's open availability and integration with popular platforms make it accessible for developers, researchers, and enthusiasts alike.
Conclusion
Conclusion
This 32-billion parameter reasoning model from Alibaba, called qwq 32b, has shown impressive performance in various benchmarks, rivaling even much larger models like the 671-billion parameter DeepSEER1.
The key advancements that enable this model's strong reasoning capabilities are:
-
Reinforcement Learning Optimization: The use of reinforcement learning significantly boosts the model's reasoning abilities, making a smaller model more intelligent.
-
Foundation Model Pre-training: The model is built on extensive world knowledge, providing a strong base for reasoning.
-
Agent-like Capabilities: The model can think critically, use tools, and adapt its reasoning based on environmental feedback.
Through our own testing, the model demonstrated strong performance in areas like mathematical reasoning, coding, and logical problem-solving. While it struggled with a more complex SVG generation task, it excelled in most other areas.
Overall, this model showcases the potential of scaling reinforcement learning and foundation model pre-training to create powerful reasoning models with relatively smaller parameter counts. It will be interesting to see how this technology evolves and how it compares to other cutting-edge AI models in the future.
Часто задаваемые вопросы
Часто задаваемые вопросы