Deepseeks' AI Self-Improvement Breakthrough Explained
Deepseek's AI self-improvement breakthrough: A novel approach to building adaptable AI models that can refine their own judgments, outperforming larger models. Unlock the secrets behind this cutting-edge innovation.
2025년 4월 10일
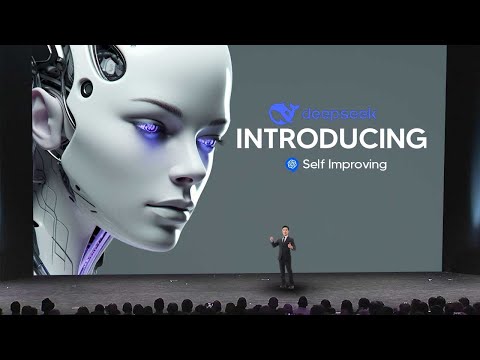
Unlock the power of self-improving AI with Deepseek's groundbreaking research. Discover how their innovative approach to AI model training can lead to remarkable advancements in performance and versatility, potentially reshaping the future of artificial intelligence.
The Promise of Self-Improving AI: Deepseek's Breakthrough
Explaining the Deepseek GRM Approach
Empowering the AI Judge with Inference Time Scaling
Outperforming Larger AI Models with Smart Combining
The Potential Impact of Deepseek R2 on the AI Industry
The Promise of Self-Improving AI: Deepseek's Breakthrough
The Promise of Self-Improving AI: Deepseek's Breakthrough
Deepseek, the renowned open research company, has recently unveiled a groundbreaking paper on self-improving AI models. The key innovation lies in their approach to training a versatile and adaptable "judge" AI, known as the Generative Reward Model (GRM), which can accurately evaluate the performance of language models across a wide range of tasks.
The GRM is trained using reinforcement learning, where it practices generating detailed principles and critiques to assess the quality of model responses. This training process teaches the GRM to produce accurate judgments that become increasingly refined over time. Crucially, the GRM's performance can be further enhanced through "inference time scaling," where the model is asked to evaluate the same input multiple times, and the results are combined using a meta-model that selects the most reliable critiques.
This approach has yielded impressive results, with the GRM outperforming larger language models like GPT-4 when used as a judge, even when the latter is only asked to evaluate once. The ability of the GRM to provide detailed reasoning for its judgments, combined with its capacity for self-improvement, makes it a powerful tool for advancing the state of the art in language AI.
The implications of Deepseek's breakthrough are significant. It paves the way for the development of self-improving AI systems that can continuously refine their capabilities, potentially leading to rapid advancements in areas such as natural language processing, question-answering, and creative problem-solving. As the industry eagerly awaits the release of Deepseek's next-generation model, R2, this research represents a significant step forward in the quest for truly intelligent and adaptable AI systems.
Explaining the Deepseek GRM Approach
Explaining the Deepseek GRM Approach
The key aspects of the Deepseek GRM (Generalized Reward Modeling) approach are:
-
Versatile Judge AI: Deepseek developed a judge AI that can provide detailed reasoning and principles behind its judgments, rather than just outputting a score. This makes the judge more flexible and adaptable across different tasks.
-
Reinforcement Learning: The judge AI is trained using reinforcement learning, where it practices generating principles and critiques, and receives rewards for judgments that match the correct ones. This teaches the judge to produce high-quality assessments.
-
Inference Time Scaling: To improve the judge's accuracy, Deepseek uses an "ask multiple times" strategy, where the judge is queried multiple times (e.g., 8 or 32 times) during inference. The results are then combined, either through voting or using a separate "meta-RM" model that evaluates the quality of each critique.
-
Outperforming Larger Models: The medium-sized Deepseek judge AI, when using the inference time scaling approach, was able to outperform much larger models like GPT-4 when used as a judge, even when those larger models were only queried once.
-
Potential for Deepseek R2: The success of the GRM approach suggests that it could be a key component of Deepseek's upcoming R2 model, which is expected to be a significant advancement in the AI field and potentially challenge the dominance of models from companies like OpenAI.
Empowering the AI Judge with Inference Time Scaling
Empowering the AI Judge with Inference Time Scaling
The key to Deepseek's self-improving AI lies in their approach to the AI judge, or the "reward model" as it's referred to in the paper. Deepseek has developed a novel solution called the Deepseek GRM (Generalized Reward Model), which addresses the limitations of traditional AI judges.
Unlike simple scoring systems, the Deepseek GRM writes out detailed reasoning and principles to explain its judgments. This flexibility allows the judge to generate slightly different critiques and scores when evaluating the same input multiple times. Deepseek then employs reinforcement learning to train the judge, rewarding it for generating high-quality principles and critiques that lead to accurate final judgments.
The real innovation, however, comes from Deepseek's use of "inference time scaling." Instead of relying on a single judgment, the system asks the GRM judge multiple times (e.g., 8 or 32 times) and combines the results through a voting process. This is further enhanced by a small "meta-RM" AI that quickly evaluates the quality of each critique, allowing the system to prioritize the best ones.
The result is a highly capable and versatile AI judge that can outperform much larger models, such as GPT-4, when used for evaluation tasks. Crucially, this performance advantage grows as the system is allowed more computational resources to sample the judge multiple times during inference.
This approach to empowering the AI judge is a key component of Deepseek's self-improving AI capabilities, and it is likely to be a central feature of their upcoming R2 model, which is expected to push the boundaries of AI performance even further.
Outperforming Larger AI Models with Smart Combining
Outperforming Larger AI Models with Smart Combining
The key to Deepseek's approach is their use of a "Generative Reward Model" (GRM) as the AI judge. Unlike traditional judges that simply output a score, the GRM writes out detailed reasoning and principles to explain why an answer is good or bad. This flexibility allows the GRM to generate slightly different critiques and scores when evaluating the same input multiple times.
Deepseek then leverages this by "sampling" the GRM multiple times (e.g. 8 or 32 times) and combining the results. This "smart combining" is further enhanced by training a small "meta-RM" model to quickly evaluate the quality of each written critique from the main GRM. Only the critiques deemed high-quality by the meta-RM are used to compute the final judgment.
This multi-step process, while computationally intensive, dramatically improves the accuracy of the final judgment compared to simply asking the GRM once. In fact, the medium-sized GRM model was able to outperform much larger AI models like GPT-4 when used as a judge, as long as the larger models were only queried once.
The key advantages of Deepseek's approach are:
- The GRM's ability to provide detailed, flexible critiques rather than just a score.
- The performance boost from sampling the GRM multiple times and combining the results.
- The meta-RM's ability to identify the most reliable critiques to include in the final judgment.
This innovative technique allows a moderately-sized AI system to achieve top-tier performance when used as a judge or evaluator, outclassing even much larger models. It represents a significant advancement in the field of self-improving AI systems.
The Potential Impact of Deepseek R2 on the AI Industry
The Potential Impact of Deepseek R2 on the AI Industry
Deepseek's upcoming release of their R2 model could have a significant impact on the AI industry. As an innovative open-source company, Deepseek has been pushing the boundaries of AI research and development. The recent paper they published on self-improving AI models showcases their ability to create advanced AI systems that can continuously enhance their own performance.
The key aspects of Deepseek's approach that could disrupt the industry include:
-
Versatile and Adaptive AI Judge: Deepseek has developed a Generalized Reward Model (GRM) that can provide detailed reasoning and critiques for evaluating AI responses across a wide range of tasks. This flexible and self-improving judge could outperform larger AI models like GPT-4 when used for evaluation.
-
Inference Time Scaling: By allowing their AI judge to sample and combine multiple judgments during inference, Deepseek has found a way to dramatically improve the accuracy of their model without the need for significantly more computational resources.
-
Potential Superiority over Existing Frontier Models: Industry experts suggest that Deepseek's R2 model could potentially outperform the latest models from other leading AI companies, such as Meta's Llama 4, which has faced criticism for its performance issues.
The release of Deepseek R2 could put significant pressure on other AI companies to keep up with the pace of innovation. It may force them to re-evaluate their own research and development strategies, potentially leading to a new wave of advancements in the field of AI. Additionally, the open-source nature of Deepseek's work could inspire and empower the broader AI community to push the boundaries of what is possible.
Overall, the potential impact of Deepseek R2 on the AI industry cannot be overstated. As the company continues to innovate and push the limits of AI capabilities, it could reshape the competitive landscape and drive the entire industry forward.
자주하는 질문
자주하는 질문